Accelerating Materials Discovery and Design with Machine Learning
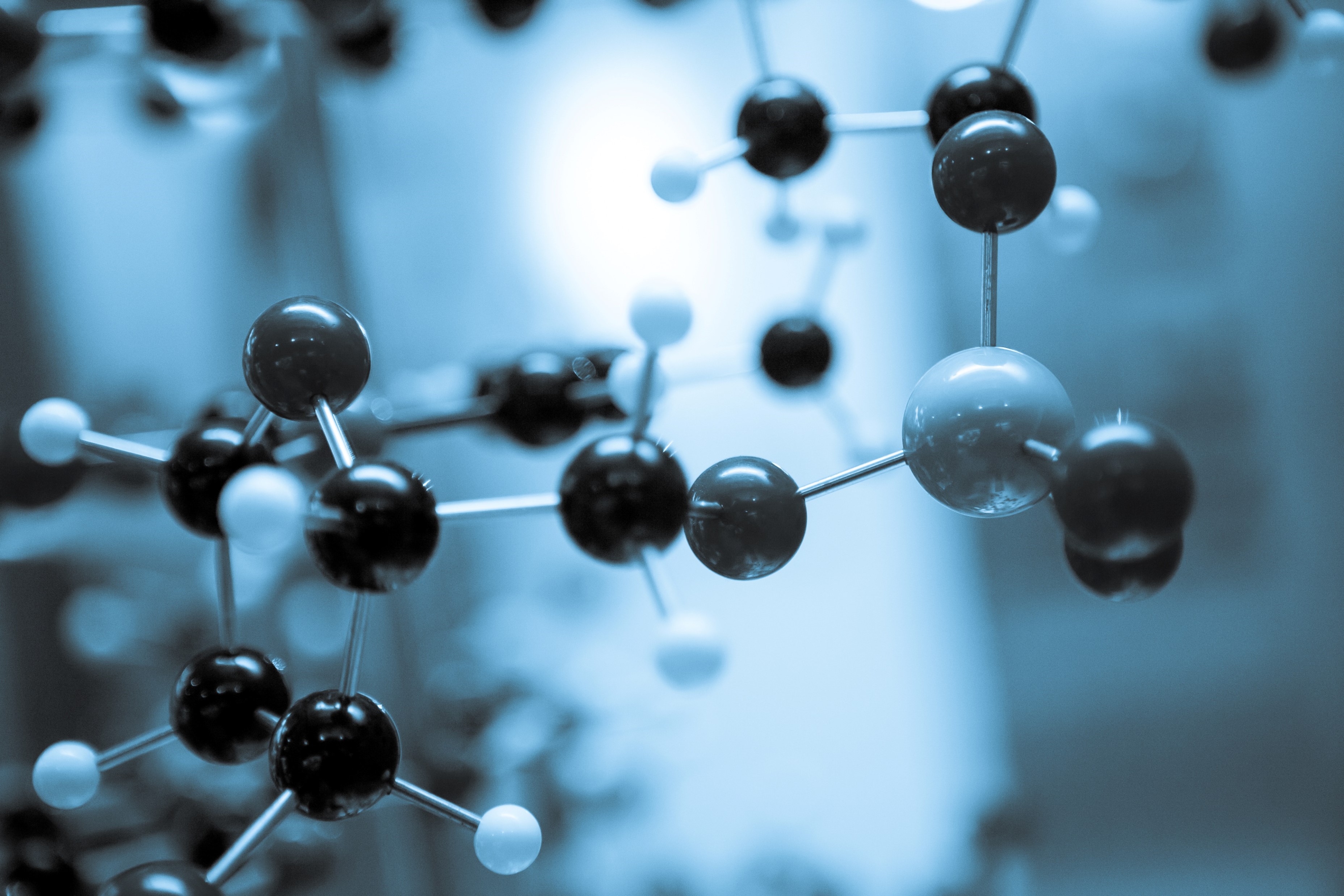
Published on 23/01/2024
Research in materials science is fundamental for developing better technologies in many sectors. Whether it is for designing higher capacity batteries, more efficient photovoltaic devices, better catalysts, or new transparent conducting oxides, plenty of novel materials and compounds are required. Numerical simulations, combined with machine learning, can help to greatly accelerate the search for the best materials to achieve these goals. This is exactly the area of expertise of Matgenix, a young company that offers services to industrial clients active in materials science and chemistry. Their goal is to numerically determine the properties of a large number of materials, based on target properties, without having to synthesize them.
Testing thousands of materials virtually is not easy
When thousands of materials need to be tested, it’s not necessary, or even possible, to synthesize them. Dr Matthew Evans, is a BEWARE research Fellow at UCLouvain and works jointly with Matgenix. He explains that at Matgenix, they virtually test those materials and suggest the most promising ones for more expensive experimental validation. However, numerical simulations are not miraculous:
Matthew Evans : “The space is too large to virtually screen all possibilities, and for some applications, calculations of target properties are extremely computationally intensive.”
To simplify this arduous task they develop and extend open-source software that enables high-throughput calculations that can be performed on hundreds of thousands of materials, real and hypothetical, that exist in open databases.
Matthew Evans : “It allows us to rapidly screen for the optimal solutions for a given application and also to robustly suggest which hypothetical materials may have the targeted properties.”
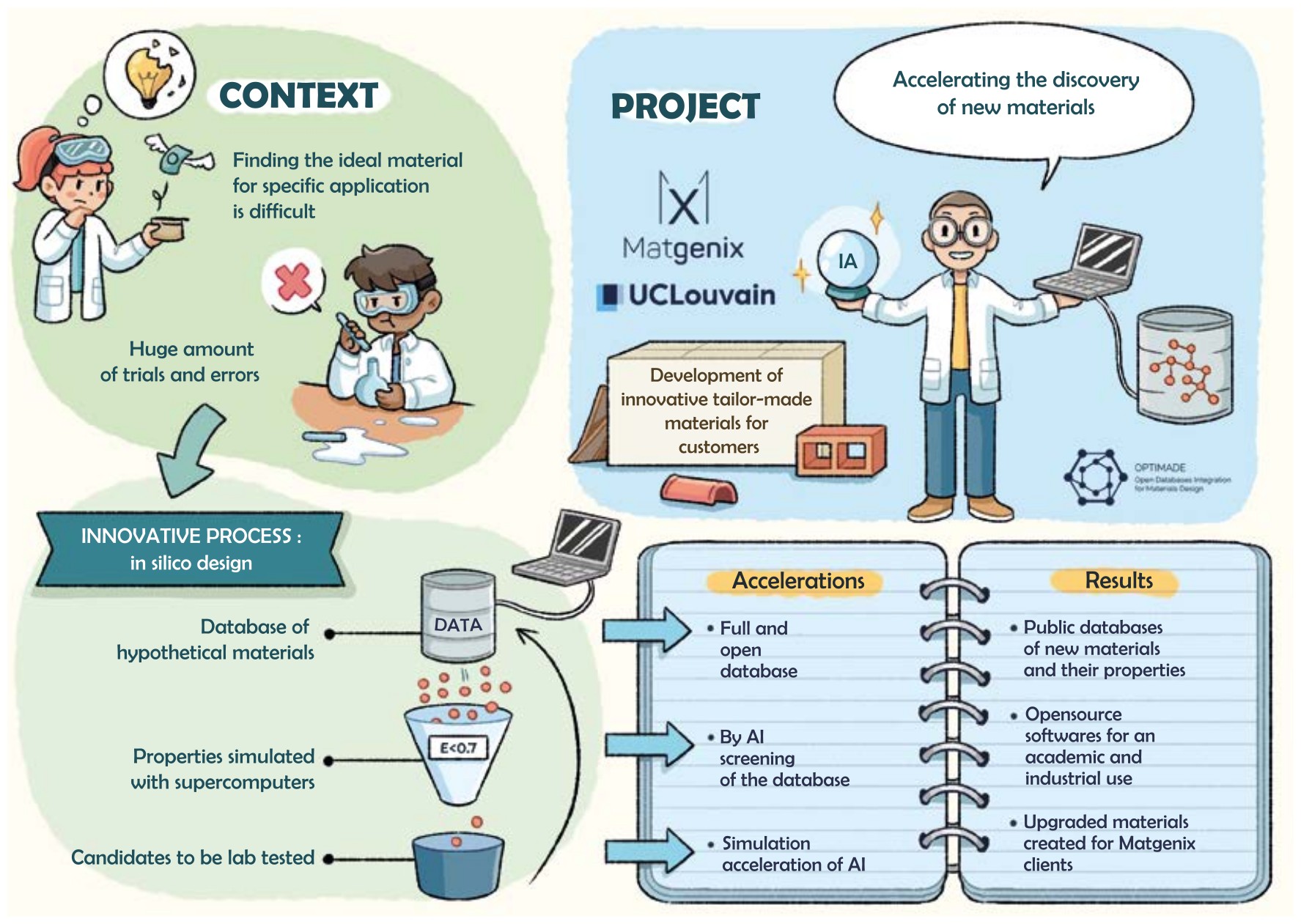
Going further with machine learning
Machine learning is also used to accelerate these steps; either by training a surrogate model that can suggest which of the thousands of potential materials to study in detail, or by training models on a particular set of materials that can predict the targeted properties with sufficient accuracy much more efficiently than traditional first-principles calculations. The inclusion of machine learning-based approaches can also allow for significantly larger system sizes to be studied in detail, providing a much better match to the real physical system.
Matthew Evans : “This combination of high-throughput automated jobs and machine learning approaches allows for unprecedented scope and scale of materials design and discovery.”
Moreover, the sizeable datasets created can then be repurposed for further machine learning studies, accelerating future developments by generating surrogate models that circumvent the need for further calculations.
Using Tier-1 and Tier-0 supercomputers
Such heavy numerical computation on thousands of trial materials requires significant computational resources. Fortunately, as the calculations for each material are independent, using the software developed by Matgenix, they can be run simultaneously, and thus benefit from some of the largest supercomputers available in Belgium, which reduces the time required to study thousands of materials to the time taken to study just one.
Matthew Evans : “The specialist compute hardware in Walloon region’s new “Lucia” machine, and in the European Tier-0 machine “LUMI” allows for many thousands of trial materials to be studied in a given day, allowing for much faster iteration on ideas and exhaustive sampling of materials space.”
A real benefit for the industry
The services that Matgenix offers are of first interest for the industry:
Matthew Evans : “Being able to virtually test materials candidates provides a significant competitive advantage over traditional trial-and-error approaches, allowing companies and researchers to explore many more ideas with greater fidelity for the same or even lower cost.”
Moreover, Matgenix also leans heavily on the robust automation of calculations.
Matthew Evans : “Combined with sizeable HPC resources, this means that the time taken to reach a reliable and systematic answer is considerably shorter than it would be otherwise.”
If you’re interested in their services, visit their website at www.matgenix.com
Dr Matthew Evans, BEWARE Research Fellow at UCLouvain & Matgenix.
Originally from the UK, Matthew studied Theoretical Physics at the University of Manchester before completing a PhD at the University of Cambridge. During this time, he used high-throughput calculations to investigate the structures formed during charging of battery electrodes. He also developed tools for combining materials data from various databases as part of the OPTIMADE consortium. During the pandemic, he then joined UCLouvain where he extended his work to use machine learning predictions, before receiving a BEWARE Fellowship from the Walloon region to work jointly with Matgenix to apply this work to more industrial applications.